Waves in North Sea better predicted by Machine Learning
In coastal engineering projects and ship navigation, predictions of waves are crucial. The installation of an offshore wind turbine in the North Sea, for example, depends on a complex interplay of wave movements, influenced by wind and swell. The more accurate and reliable wave forecasts are, the better the execution of projects at sea can be planned. Experts from Coastal Structures & Waves at Deltares discovered that you can better predict wave movements by linking process-based models to Machine Learning models.
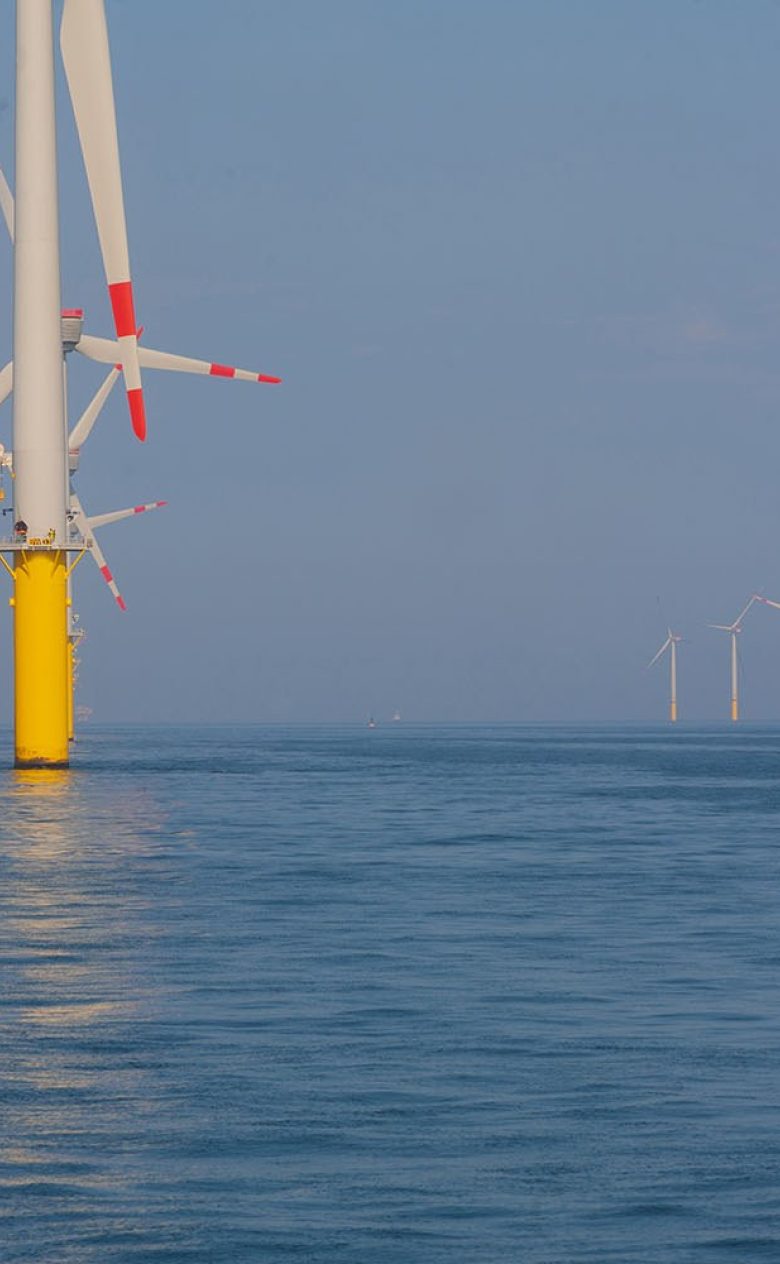
Joost den Bieman, senior researcher at Coastal Structures & Waves and one of the experts on this study, explains that fast, accurate wave forecasts provide valuable information for navigation, coastal protection and offshore structures. "This is because it allows you to more accurately plan your workable days and predict tidal windows for ships. Fast, accurate data means a better workflow and less risk of damage to installations and ships. So that's profit!", says Joost.
Complex patterns
Traditionally, process-based models are used to simulate wave motions. These models are based on physical principles of the interaction between wave propagation and seabed bathymetry. "These existing models provide very detailed information about waves. But, they also have their limitations," says Joost. "To get the wave predictions in the North Sea significantly better, we combine them with Machine Learning models." These have enabled huge advances in science and technology in recent years. By training Machine Learning models on historical wave data, they learn to recognise complex patterns from large data sets. With this, they can supplement shortcomings of traditional process-based models. "Especially in places where physics is difficult to model," Joost explains.
Best of both worlds
"The aim of our research is to combine the best of both worlds," says Joost. The team of experts used hybrid modelling for this purpose. In the test phase of their research, they performed a clever integration of both models: the accurate physical principles of the process-based models as well as the strong prediction power of the Machine Learning models. "These models can identify complex trends and relationships in large datasets, which are not always easy to model with traditional, physical models," Joost explains. "We train the Machine Learning model with an extensive dataset of historical wave observations and forecasts."
Results
To validate and evaluate the hybrid model, the team conducted extensive testing with different datasets. To this end, the performance of the hybrid model was compared with that of individual process-based models. The team also compared the predictions of the hybrid model with observed data to assess its accuracy and reliability. The hybrid model was compared with the individual SWAN model. This is a type of wave model used for the North Sea, among others. These experiments show that the hybrid model has superior performance. This model makes about 30% to 40% fewer errors in the forecast," says Den Bieman. "This significantly increases the accuracy of operational wave forecasts with a time horizon of 48 hours."

Promising application
According to Joost, their research shows that combining process-based models and Machine Learning models is a promising application to yield fast, reliable wave predictions. "The hybrid model allows us to gain more insight into complex wave behaviour. At the same time, the model provides fast and accurate predictions of current conditions and weather conditions." Shipping companies can use it to optimise their routes and offshore projects can be carried out more safely. Joost believes there is a need to further develop and refine the hybrid model. "Machine Learning models are data-driven, so the more relevant data available for training, the better." He points out that this also applies to the wave data set. "For example, you could 'retrain' the Machine Learning model every year. This provides an extra year of data."
Shipping companies can use it to optimise their routes and offshore projects are carried out more safely
Joost den Bieman
Significant optimisation step
Hybrid modelling is still in its infancy. According to Joost, a logical next step is to find out whether combining process-based and Machine Learning models can also add value for other applications. "In any case, the application in the North Sea shows that waves can be better predicted, which represents a significant optimisation step for maritime and coastal-related activities," Joost says.
Publication
Together with fellow researchers Menno de Ridder, Marisol Irías Mata and Joana van Nieuwkoop of Coastal Structures & Waves at Deltares, senior researcher Joost den Bieman published the scientific paper 'Hybrid modelling to improve operational wave forecasts by combining process-based and machine learning models.' in May this year. In this article, they describe in detail how waves can be accurately and reliably predicted by combining process-based models with Machine Learning models.
